Projects
Hybrid-AI Quantile Regression for Spatio-Temporal Climate Analysis
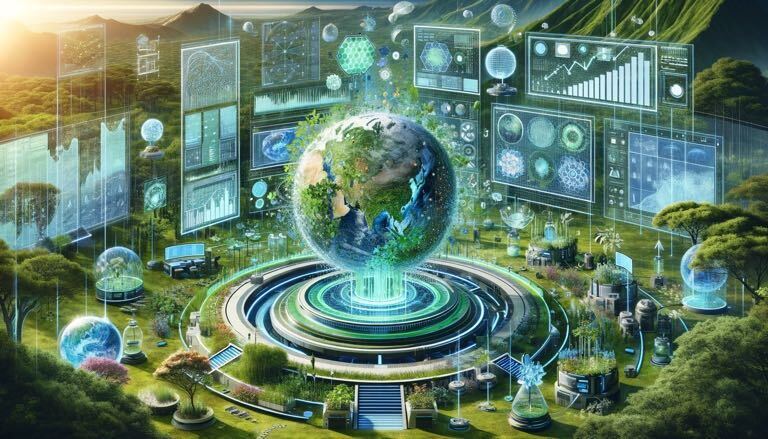
- This project develops a Hybrid-AI Quantile Regression framework, merging nonparametric statistical methods with physics-informed neural networks.
- It aims to enhance the understanding and prediction of climate phenomena by analyzing spatio-temporal climate data.
- The framework’s versatility is showcased in a case study using rainfall data from India and Israel, highlighting its potential in environmental research, urban planning, and climate change mitigation.
Advanced Statistical Inference for Dynamical Systems
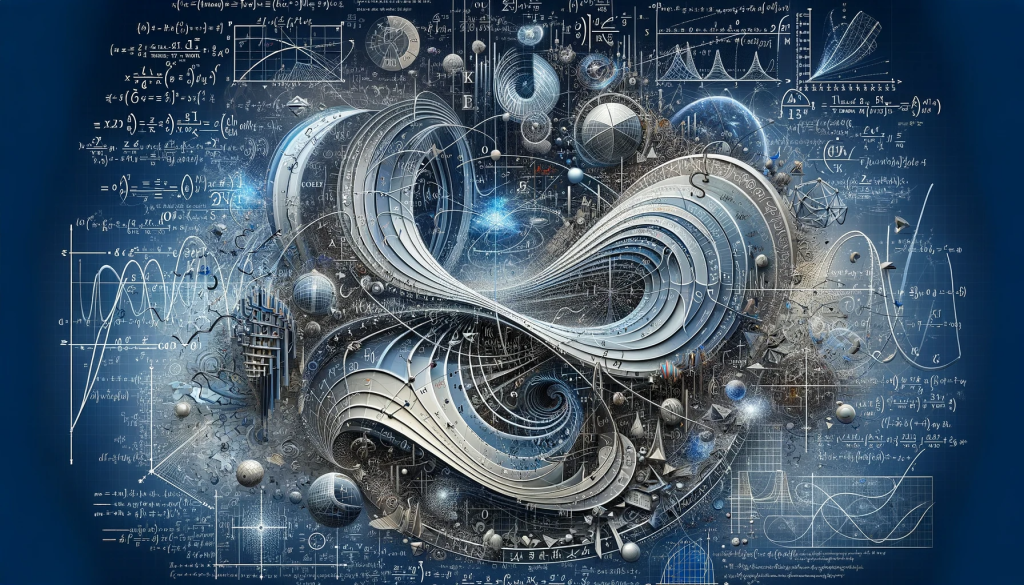
- This project aims to develop innovative methods for model selection and lack-of-fit testing in the context of Ordinary Differential Equations (ODEs) modeling of dynamical systems.
- Focusing on nonlinear systems, both fully and partially observed, it introduces testing methods for model selection and nonparametric testing of lack-of-fit.
- This research will have significant implications in various scientific fields, such as engineering, medicine, psychotherapy, epidemiology and biology, offering new statistical tools for understanding complex dynamical processes.
Innovative AI-Driven Approach for the Development of Postharvest Protocols Under Extreme Climatic Conditions
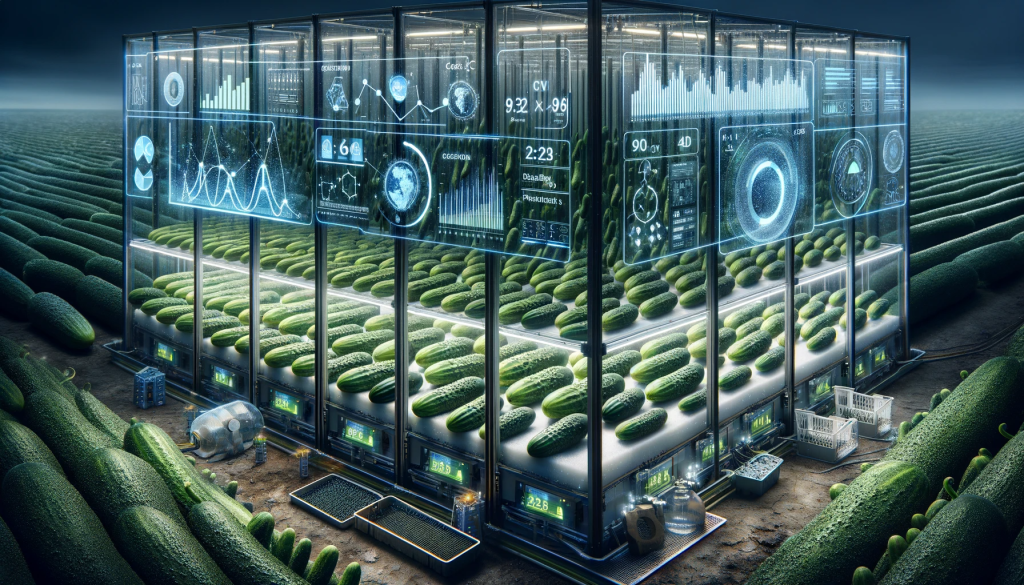
- This project focuses on the development of postharvest protocols using physics-informed machine learning for assessing the quality of cucumbers, zucchini, champignon, and portobello mushrooms under extreme climatic conditions.
- By integrating advanced AI with physics-based insights, the project aims to accurately predict produce fitness through image analysis.
- In colaboration with MIGAL – Galilee Research Institute.
Dynamical System Modeling and Statistical Inference in Psychotherapy
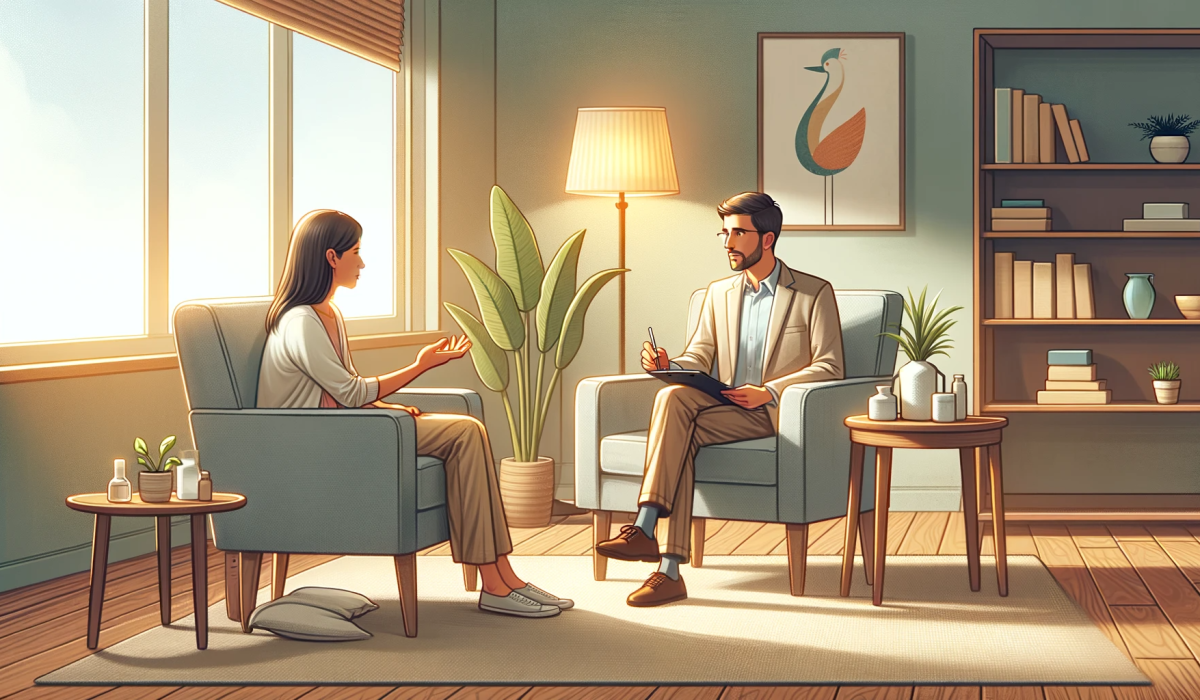
- This project applies dynamical system modeling and statistical inference to enhance understanding and efficacy in psychotherapy treatment.
- It employs mechanistic models such as Ordinary Differential Equations (ODEs) and physics-informed machine learning to delve into the complexities of psychotherapeutic processes.
- Additionally, mixed effects nonlinear modeling is utilized to capture the nuanced variations in therapy outcomes, offering a comprehensive and sophisticated approach to analyzing and improving psychotherapy practices.
Robust Optimization and Parameter Estimation in Queueing Networks
- This project pioneers advancements in optimization and optimal control within queueing systems and queueing networks.
- With a focus on both optimization under uncertainty and the estimation of model parameters, we tackle the challenges posed by systems experiencing high demand for constrained resources.
- Our efforts seek to enhance decision-making in this complex environment by refining statistical parameter estimation techniques as well as finding solutions that are optimal under the uncertain circumstances.
Investigating Verbal and Non-Verbal Synchronization in Preschool Children
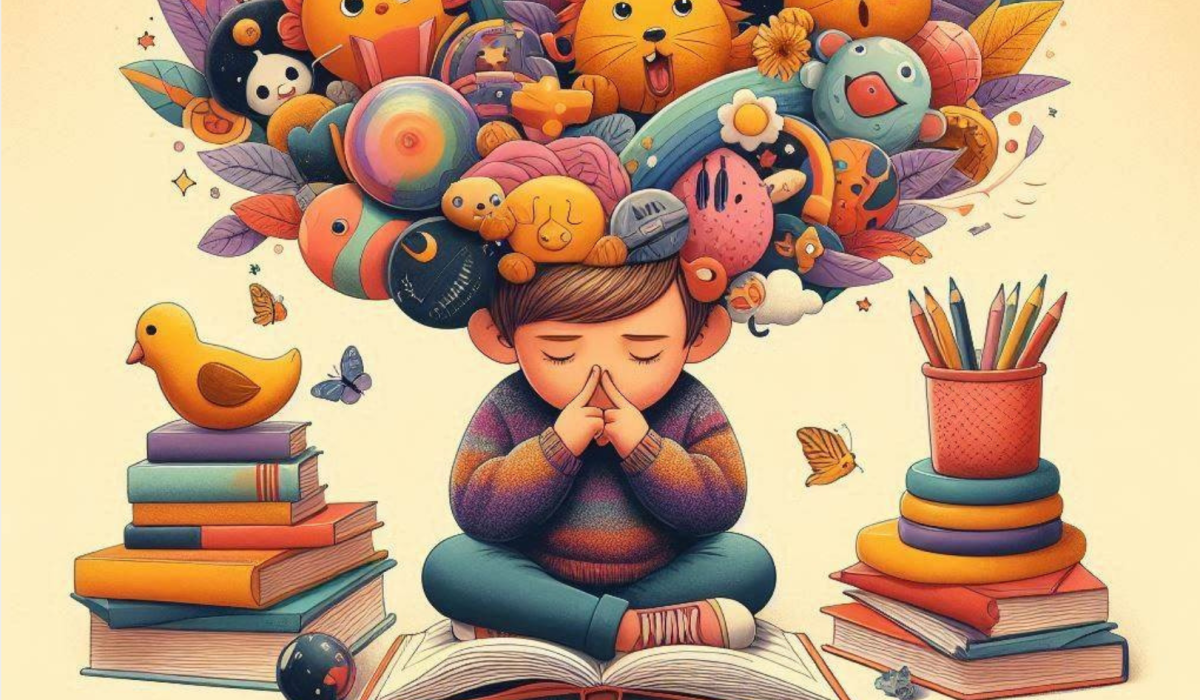
- This research presents a novel and comprehensive study aimed at understanding and enhancing communication among preschool children by examining both verbal and non-verbal synchronization.
- Verbal synchronization refers to how individuals naturally adjust their speech patterns, including accents, intonation, and vocabulary, to align with others, while non-verbal synchronization involves coordinating physical movements, such as gestures.
- The study seeks to define and measure these forms of synchronization within triads of preschool children, investigate their relationship, explore their development with age, and examine their connection to communication characteristics.
- Additionally, the research aims to develop a synchronization index to assess communication in young children, leveraging advanced AI and machine learning techniques.